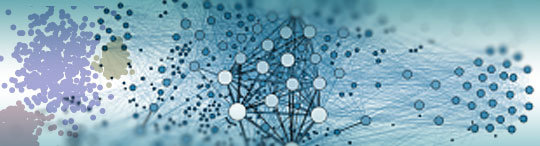
Statistical Data Analysis for Practitioners
In science, as well as in our daily lives, we are constantly confronted and dealing with uncertainties. Probability theory and statistics provide the tools to learn from uncertain information and data and to make choices and decisions in the presence of uncertainties.
Goals of the Course
The goals of the lecture are to equip the students with the necessary statistical tools to extract information from noisy data reliably and with quantified uncertainties. The students should be able to identify the common pitfalls of statistical data analysis in their own work and be able to critically assess the quality of published data and statistical analyses.
Format
Currently, the course is held as a single block on seven consecutive work days. Lectures will be mixed with practical exercises. In these exercises you will mainly apply the widely used Python programming language to perform statistical data analysis yourself.
Practical Course
The practical exercises are designed such that you need only limited programming skills. The exercises consist of Python code, which you have to modify to obtain the desired results. That is, you will choose, set, and modify parameters and select appropriate functions to analyze data. This approach mimics the ubiquitous real-world task to modify someone else’s code for your own purposes.
For the practical course, you need to bring your own laptop. The course material will be made available via Binder online, such that you only need an internet connection. Alternatively, you can use your own Python installation.
For beginners, we offer a short crash course in Python. Programming experience in any language is helpful and recommended but it is not a precondition. You can do the exercises by yourself or pair up with a partner, perhaps with more programming experience.
Lecturers
The lectures and practical course are held alternatingly by different lecturers according to their core expertise. All lecturers have extensive research experience in statistics and data analysis and teaching experience.
Prof. Dr. Roberto Covino is an independent group leader at the Frankfurt Institute for Advanced Studies (FIAS). His background is in theoretical and computational physics. His research aims at developing and applying theoretical models, computer simulations, and artificial intelligence methods to understand the emergence of complex biomolecular structures, dynamics, and functions from physical principles. He has been teaching multiple different courses at Goethe University, including a course on biomolecular simulations, membrane biology, and lectures on statistics, data analysis and machine learning.
Dr. Sergio Cruz León is a postdoctoral researcher at the Max Planck Institute of Biophysics. Sergio is a physicist by training. He is currently developing and applying methods to quantify the spatial arrangement of proteins and nucleic acids inside cells to elucidate their dynamics and function. He uses an interdisciplinary approach, integrating molecular dynamics simulations, cryo-electron tomography, and advanced statistics-based image analysis methods. He is an experienced lecturer and has taught multiple courses on fundamental physics.
PD Dr. Jürgen Köfinger is a project leader at Max Planck Institute of Biophysics and has obtained his Habilitation at the Department of Physics of the Goethe University in 2021. He is a physicist by training. Jürgen’s research interests focus on integrative modeling in general and ensemble and force field refinement in particular. In his research, he routinely applies probability and information theory, Bayesian inference, and maximum entropy methods. He has been teaching multiple different courses at Goethe University since 2015 and has been giving this lecture on statistics and data analysis yearly since 2017.
Dr. Karen Palacio-Rodriguez, a chemist by training, is currently a postdoctoral researcher at the Max Planck Institute of Biophysics. Her research focuses on elucidating the mechanical properties of large biomolecular complexes through physics-based modeling. In her research, she has been developing methods for estimating thermodynamic and kinetic properties from molecular dynamics simulations. Karen has systematically applied and developed likelihood maximization frameworks and statistical tests to accurately recover dynamics from out-of-equilibrium simulations.
Content
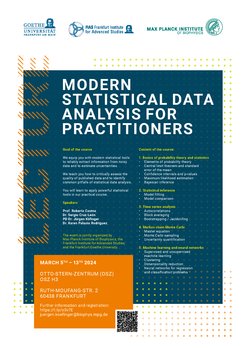
Basics of probability theory and statistics
- Elements of probability theory
- Central limit theorem and standard error of the mean
- Confidence intervals and p-values
- Maximum likelihood estimation
- Bayesian inferenceStatistical inference
- Model fitting
- Model comparisonTime series analysis
- Autocorrelations
- Block averaging
- Bootstrapping / JackknifingMarkov chain Monte Carlo o Master equation
- Monte Carlo sampling
- Uncertainty quantificationMachine learning and neural networks
- Supervised and unsupervised machine learning
- Clustering
- Dimensionality reduction
- Neural networks for regression and classification problems